CSC News
What Gamers Want: Researchers Develop Tool To Predict Player Behavior
For Immediate Release
Matt Shipman | News Services | 919.515.6386
Dr. David L. Roberts | 919.513.7182
Researchers from North Carolina State University have developed a new method that can accurately predict the behavior of players in online role-playing games. The tool could be used by the game industry to develop new game content, or to help steer players to the parts of a game they will enjoy most.
“We are able to predict what a player in a game will do based on his or her previous behavior, with up to 80 percent accuracy,” says Brent Harrison, a Ph.D. student at NC State and co-author of a paper describing the research. The research team developed the data-driven predictive method by analyzing the behavior of 14,000 players in the massively multiplayer online role-playing game (MMORPG) World of Warcraft.
“In a game like World of Warcraft, which is constantly developing new content, this could help guide content design decisions,” Harrison says.
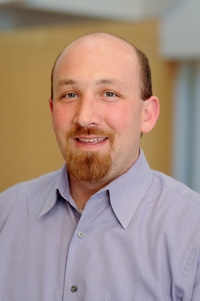
“This work could obviously be used for World of Warcraft or other MMORPGs,” says Roberts, “but it also applies to any setting where users are making a series of decisions. That could be other gaming formats, or even online retailing.”
Harrison adds that the new methodology could also help game designers guide players to existing content that is suited to their gaming style.
“For example,” Roberts says, “you could develop a program to steer players to relevant content. Because it is a data-driven modeling approach, it could be done on a grand scale with minimum input from game designers.”
The researchers developed the new method by evaluating the task-based “achievement” badges that players in World of Warcraft earn. These achievements are awarded whenever a player accomplishes a specific goal or series of goals.
Specifically, the researchers collected data on 14,000 players and the order in which they earned their achievement badges. The researchers then identified the degree to which each individual achievement was correlated to every other achievement. The researchers used that data to identify groups of achievements – called cliques – that were closely related. Those cliques could then be used to predict future behavior. For example, if a clique consists of seven achievements, and a player has earned four of them, the researchers found that they will probably earn the other three. However, many of the cliques that the researchers identified consist of 80 or more different achievements.
One interesting element of these findings is that the achievements that are highly correlated – or part of the same clique – do not necessarily have any obvious connection. For example, an achievement dealing with a character’s prowess in unarmed combat is highly correlated to the achievement badge associated with world travel – even though there is no clear link between the two badges to the outside observer.
The paper, “Using Sequential Observations to Model and Predict Player Behavior,” will be presented at the Foundations of Digital Games Conference in Bordeaux, France, June 29-July1.
NC State’s Department of Computer Science is part of the university’s College of Engineering.
-shipman-
Note to Editors: The study abstract follows.
“Using Sequential Observations to Model and Predict Player Behavior”
Authors: Brent Harrison and David L. Roberts, North Carolina State University
Presented: June 29-July 1, 2011 Foundations of Digital Games Conference, Bordeaux, France
Abstract: In this paper, we present a data-driven technique for designing models of user behavior. Previously, player models were designed using user surveys, small-scale observation experiments, or knowledge engineering. These methods often produced questionable models or were too narrowly focused to be of general use. To address these issues, we have developed a purely data-driven methodology for generating player models based on past observations of other players. Our underlying assumption is that we can accurately predict what a player will do in a given situation if we examine enough data from former players that were in similar situations. We have chosen to test our method on achievement data from the MMORPG World of Warcraft. Experiments show that our method greatly outperforms a baseline algorithm in both precision and recall, proving that this method can create accurate player models based solely on observation data.
Return To News Homepage